The article last week discussed how we have digitalized enquiry capture and lead nurturing through Accelerator (TVS-Accelerator-One-Powerful-App-For-Managing-All-Customer-Enquiries). Over the following few articles, we will highlight how we combine AI with digital technologies in enquiry conversion to power customer experience and business performance.
Background
At TVS Motor Company, we see large volumes of consumers interested in buying two-wheelers. Such enquiries have different propensities to buy as they may be at different stages in their buying journey. Each lead creates the need for the TVS Motor marketer and dealer sales staff to follow up promptly and personalised. Since the lead time to purchase is typically between 0-90 days, it is essential to consider the potential purchase timing while following up. At any point, our dealer partners in India will have 5+ lakh enquiries to follow up. This creates two challenges:
- Consumers with a high propensity to buy may not get followed promptly.
- There may not be sufficient ROI to follow up on all leads without considering possible time to buy.
Data sciences
Such business problems are a natural fit for data sciences. The team took on building a suite of lead classification models to predict the propensity to buy of individual consumers to help the sales staff follow up on a timely basis. Further, this would help the marketing teams activate a personalized nurturing strategy for prospects.
Solution approach
We followed the TVSM way of data sciences to tackle this problem:
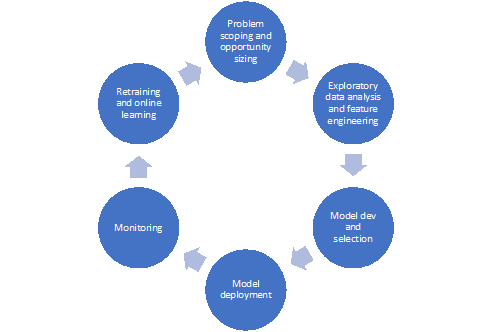
Problem scoping
We get enquiries from multiple sources such as digital campaigns, direct walk-ins and partner websites. Enquiries from these sources have different conversion rates. Also, the underlying data quality and features are very different across the sources; hence we decided to go ahead with a suite of machine learning models.
Since the objective is to enable dealer sales staff through Accelerator, our enquiry management system and given the use cases for marketing/media teams, we made two decision choices:
- Apart from the individual propensities to buy, cluster the leads based on similar propensities to hot, warm and cold categories for ease of internalization amongst our distributed chain.
- Enable a multi-source, multi-consumption platform through the following engineering architecture.
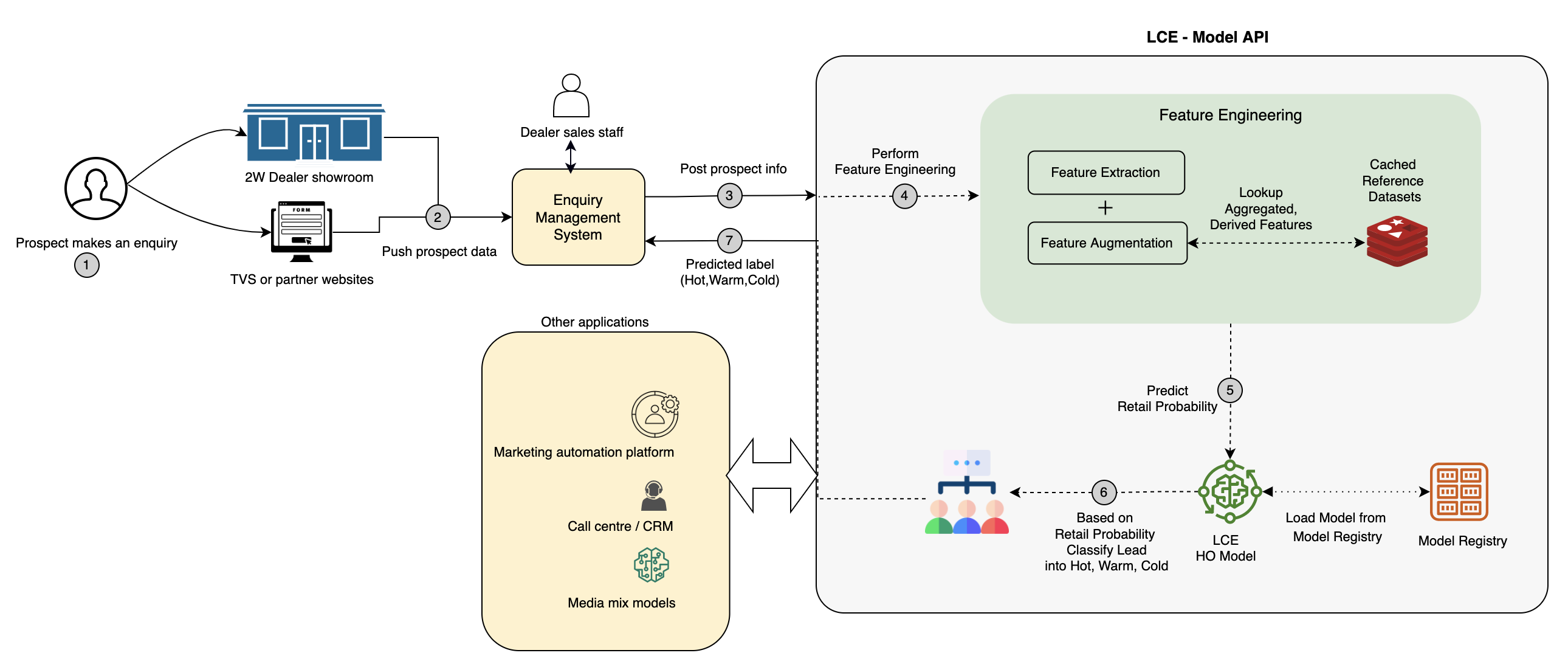
As seen in the above architecture workflow, the Lead Classification Engine (LCE) model has been hosted on the Azure cloud and exposed as a REST API. Whenever the Enquiry Management System (EMS) receives a lead, it immediately calls LCE Model API to score it in real-time. It computes the retail probability, classifying it into hot, warm or cold. Other marketing and CRM applications can also consume the predictive labels since the LCE model is hosted as REST API.
Opportunity sizing
To estimate the ROI from the model, it was essential to baseline the current event rate against the estimated lift in the event rate. The charts below show the event rate* (Y-axis) across various enquiry sources and projected event rates. The X-axis indicates the number of days from the day of enquiry creation over with conversion events taking place.
Cumulative event rate (Existing vs Projected) over 90 days
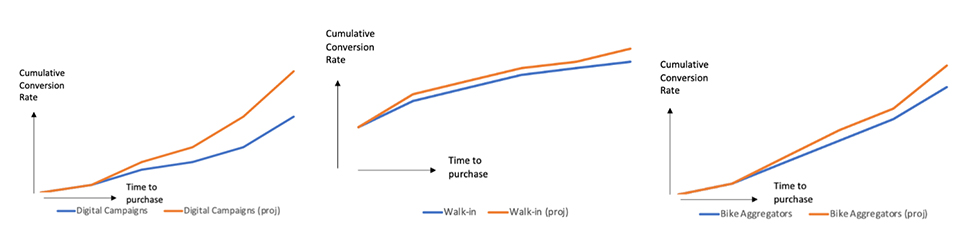
*All numbers are illustrative as these are company confidential information
The above baselining exercise allowed us to have a ballpark sense of the impact our models could deliver. By classifying the leads based on propensity to buy and with appropriate, personalized follow-up actions, there was a significant business opportunity to improve customer experience and generate additional revenue.
The following few articles will continue this discussion and highlight how we have enabled production scale AI algorithms in enquiry nurturing and conversion.
- Algorithms: Exploratory data analyses, algorithm development and model selection
- AI ops: Deployment, monitoring and online learning
Stay tuned for more!
Please do let me know how to access this session.